Artificial intelligence (AI) is a discipline that has come 70 years down the road in the history of computer science. Its genesis dates back to the 1950s, when pioneers such as Alan Turing, the brilliant British scientist who played a crucial role in deciphering German messages during World War II, laid the foundations of this novel branch.
In his 1950 essay, entitled “Computing machinery and intelligence”, Turing ventured a fascinating prediction: that in about fifty years we could be talking about thinking machines. Five years later, John McCarthy, an American mathematician, coined the term “artificial intelligence” to describe this new discipline.
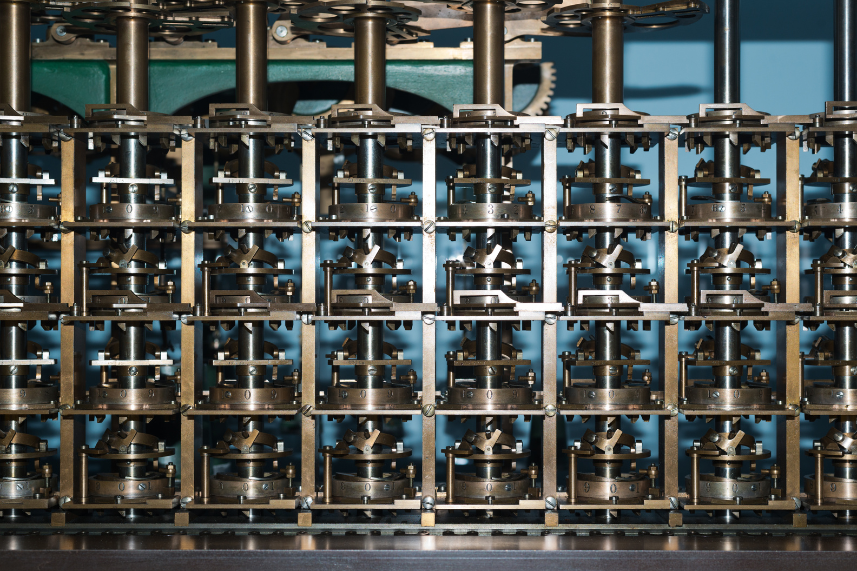
The evolution of AI has been anything but uniform, marked by periods of intense activity and remarkable advances, interspersed with periods of skepticism and stagnation, known as the “winters of artificial intelligence”. Turing, in his 1950 essay, posed the fundamental question: Can machines think? But instead of answering it directly, he proposed the famous “Turing Test,” a game in which a questioner must decide which of two interlocutors, one human and one machine, is the human being after a five-minute conversation. If the machine fooled the interrogator, it was considered to have passed the test by demonstrating human or at least human behavior.
During the 1960s and 1970s, despite the computational limitations of the time, remarkable advances were made, such as the creation of the first functional chatbot called “Eliza” and the development of artificial neural networks with backpropagation, a fundamental algorithm in supervised learning. This era also saw the emergence of interest in machine translation.
However, between 1975 and 1980, what is considered the first “AI winter” was experienced. Expectations around areas such as machine translation, which had aroused great interest due to their potential usefulness in the Cold War, faded. The use of perceptrons, basic artificial neural networks, also encountered challenges that led to a decline in interest and funding in AI projects.
In the beginning, AI was oriented towards “strong AI” with the ambition of replicating human intelligence for application in various activities. However, in the 1980s, given the difficulty in achieving this goal, the focus shifted to “weak AI”, focusing on specific applications such as financial planning, medical diagnostics, computer vision and other specialized fields.
The second “AI winter” occurred between 1985 and 1995, characterized by apathy in research and investment due to disappointing results from programs around the world. Many companies went bankrupt and expert systems, which had been a promise, received criticism from experts, including a prominent critique from John McCarthy, the mathematician who coined the term “artificial intelligence.”
The late 20th and early 21st centuries saw significant advances, driven by improvements in computational power and specific engineering methods. In 1997, the Deep Blue computer, developed by IBM, defeated world chess champion Garry Kasparov, marking an important milestone. In the same year, two Stanford University students created Google, a company that would revolutionize AI for years to come. Also notable was the U.S. agency Darpa’s autonomous car race in 2005, where five vehicles successfully completed a 212-kilometer course. In this period, essential tools such as the Python programming language and the open-source numerical computation library Numpy emerged.
In 2004, Google published a paper that popularized the programming model, inspiring the creation of Hadoop in 2006, an open source system for analyzing large amounts of data, ushering in the era of Big Data. The combination of massive data availability, reduced infrastructure costs and increased computational capacity was a key turning point. These factors enabled the industrialization of advanced machine learning techniques and the development of increasingly complex neural networks.
During this period, technological milestones occurred in specific fields of weak AI, including the emergence of IBM Watson, the passing of the Turing test by a machine pretending to be a teenager in 2014, the rise of virtual reality, and the launch of Amazon’s Alexa virtual assistant in 2014. Thanks to the growth of open source, software libraries such as TensorFlow and XGBoost emerged, enabling the capabilities of machine learning algorithms to be harnessed. Data analytics communities, such as Kaggle, became popular, where information is shared and people compete to find the best algorithms to solve business-funded problems.
The ability to solve any problem, rather than just a specific one, is known as general intelligence or “AGI”. AI researchers argued in the early 2000s that AI development had largely abandoned the field’s original goal of creating artificial general intelligence. The study of AGI was established as a separate sub-discipline and there were academic conferences, labs and university courses devoted to AGI research, as well as private consortia and start-ups, by 2010.
General artificial intelligence is also referred to as “strong AI”, “full AI” or a type of synthetic intellect rather than “weak AI” or “narrow AI”.Even if the benefits of AI are not always obvious, it has proven to be able to improve process efficiency, decrease errors and manpower, and extract information from big data.
About AlgoNew
At AlgoNew, we add intelligence to your digital interactions so you can deliver a personalized and efficient experience to your customers. How do we do it? Through a combination of intelligent decision management, natural language processing and advanced analytics.
We use algorithms to help you make informed decisions in real time and improve the efficiency of your processes. In other words, we make sure that every action you take is based on relevant data and artificial intelligence, resulting in faster and more accurate decision making.
Conversation management, on the other hand, refers to how you interact with your customers through digital platforms such as chatbots or virtual assistants. We use natural language processing technology to understand and respond to customer requests in an effective and natural way. This means that your customers can interact with digital systems in the same way they would interact with a human, which enhances the user experience.
Finally, we use advanced data analytics to gain valuable insights from your digital interactions. We analyze the data generated from your interactions to identify patterns and trends that can help you improve your business. This can include things like identifying common problems your customers have and how to solve them efficiently, or identifying areas for improvement in your business processes.
This combination of intelligences that we offer at AlgoNew can help you significantly improve your digital interactions with customers. It helps you make informed, data-driven decisions, interact with them in an effective and natural way, and gain valuable insights into your business processes.
It all leads to a better customer experience and greater business efficiency!